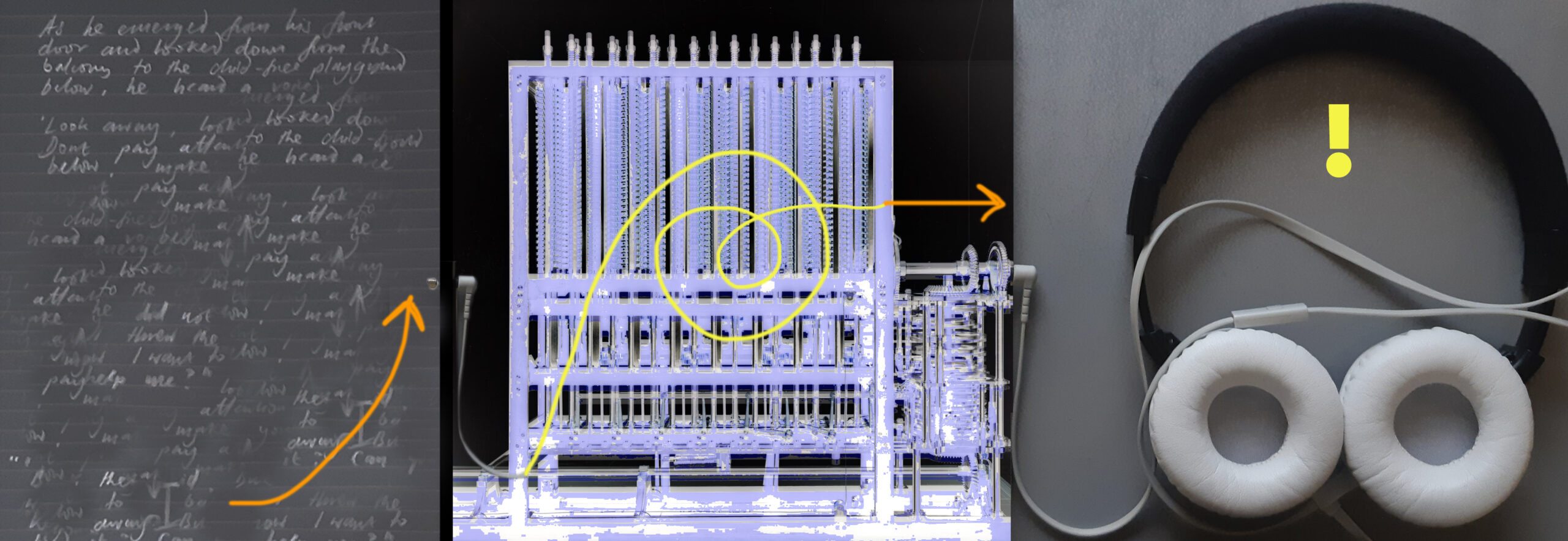
The Big Reveal
AI as a new space for creative writing and audio experience.
Imagine an audience member at point A, wearing headphones, listening to pre-scripted narration that reveals creative spirits at large – talking to listeners, drawing them into a story. Other scripted text awaits at locations B, C, D etc.
The user moves unpredictably between them – as they do, AI generates spoken text that bridges the story from one place to another, regardless of the route, with compelling narrative traction. The thresholds between AI and not-AI may be undetectable, or may announce themselves, like doors to a different realm…
The Big Reveal (WT) is a project researching how to make this possible, bringing together colleagues from different disciplines: Tim Hopkins, Sam Ladkin, Andrew Robertson, Kat Sinclair and David Weir. We’ve had very welcome support also from Jo Walton and other colleagues, and have arranged future contribution from Victor Shepardson (voice synthesis.)
AI and adaptive technology create narrative fiction in audio form, responsive to location, in AR Audio experiences for headphones and mobile devices.
New developments in generative pre-trained transformer (GPT) technology extend uses of deep learning to produce text. This is a branch of machine learning/AI that has many potential uses and societal impacts. The project explores this using AI and AR as a creative space for collaboration between engineers and artists.
We are interested in affording practitioners across these disciplines a space to learn by making something together, perceiving AI as a new space for creative writing in a multidimensional expressive space, and making something that might offer the public an engaging way to experience and reflect on their space and the growing presence and impact of AI.
The project envisages three elements, each with its own development stage.
1 a text-generation system that is adaptive to context in a way that sustains / plays with suspension of disbelief
2 voice synthesis that can translate text into convincing speech / narrative voices
3 a platform combining software which can fuse detection of user activity and location with adaptive delivery on a mobile device
Progress so far
This has focussed on 1 (as this will define scope for an application for a large project grant supporting the research phases 2 and 3).
Our method has been to develop a lab simulation combining some key technology, functionality and artistry as a proof-of-concept.
We come from different disciplines. One of the inherent stimulations for the project is navigating differences between how we conceive and share what we are trying to do. For example, David and Andrew (Informatics) have provided essential insights and guidance on current work with language models, to help induct Tim, Sam and Kat (MAH) into a complex and huge field. T, S and K have experience of writing / creating for related spaces (e.g. games, adaptive systems, branching narratives), as well as more traditional contexts, but the concepts and engineering potentially underpinning our project ask for new understandings. Similarly, discussions of language features at work in creative writing (e.g. complex implications of syntax) may test the functionality and limits of existing automated language models.
A central task has been to look for an approach that can respond significantly to what might be minimal input (prompts) from the user. In contrast to some game formats, where players’ active choices overtly steer subsequent events, we are interested in an experience where users might not perceive any instructional dialogue with a system at all, but feel as if they are being told or are immersed in a narrative that recognises what experience they are having, and is able to determine what they should hear next. This needs to happen according to a given narrative world and its bespoke generative language model – adapting to information detected by a mobile device as to location, orientation, direction, speed.
A series of discussions (April-November 2022) each led to tests based on existing text2text approaches, whereby text is put into a language model that infers suitable responses based on a range of data. Although ultimately in our user’s experience there may be no apparent text prompt from the user themselves, there is nonetheless a need for an underlying element of this kind in order to stimulate a generated response. ‘Text’ in this case may be adaptive written text users actually hear, or an equivalent input determined by their behaviour, generating ‘text’ or prompts that may be hidden from users’ perception. Our tests involved texts / prompts written by Andrew, Kat, Tim and Sam, fed through a number of text generation processes (onhttps://huggingface.co/ , a prominent platform for AI projects.)
Instead of shorter prompts leading to consequent longer responses, many of these processes were originally designed to achieve different kinds of results – such as inferring summaries of information from input texts. This tended to result in short outputs that were not quite right for our purposes in a variety of ways. Extending prompts did not flex responses more effectively. Varying the character of prompts, for example imitating strongly-flavoured genres, had some perceivable impacts, but not decisively. We needed to develop functionality towards richer responses. This suggested adjusting our approach, involving two current directions.
Current and next steps
Firstly, we continue to explore core needs – text2text generation, and training a GPT2-like model. However, we’re focussing on getting a ‘good start’ (DW) to an automated response of the kind we might want – rather than concerns about the length of response (which can be addressed later.) We are also identifying specific corpora to fine-tune a model. Andrew has been experimenting for example using ‘film reviews’ as inputs (recently using https://huggingface.co/distilgpt2.) Kat is supplying examples of poetry (including her own) and shortly larger corpora needed to train a classifier – something that can distinguish between kinds of input in the way we need. Andrew is now working on building a test model based on GPT2 to be fine-tuned with this.
Secondly, the creation of some kind of ranking machine. For example
- a) we give the same input to create a range of candidate texts (e.g.100), a machine ranks them according to merit, and randomly chooses from the top of pile
- b) we have two blocks of text – one visible one not. We insert candidate third blocks between the visible and the hidden, and rank the insertions according to how well they work between the two. (This discussion also included ‘similarity metrics’ and BERT representation – ‘Bidirectional Encoder Representations from Transformers’).
- c) we compare prompts with two corpora of texts – one has features we are looking for (e.g. of genre or form), the other is overtly more neutral (e.g. informational website like BBC news) – the machine ranks highest those closest to the first.
In the new year (23) we will pick up on these, aiming to make our proof-of-concept model in the Spring. Towards our grant application, we will also start scoping phase 2 – on voice synthesis – with input from Victor Shepardson (Iceland, Dartmouth, delayed this year due to COVID19 impacts.) We will look at challenges and consequences for translating text responses into persuasive speech simulation, and practical issues around processing – since the outcome envisages accompanying users across ‘borders’ in real time, between recorded adaptive narration and AI assisted/generated narration.
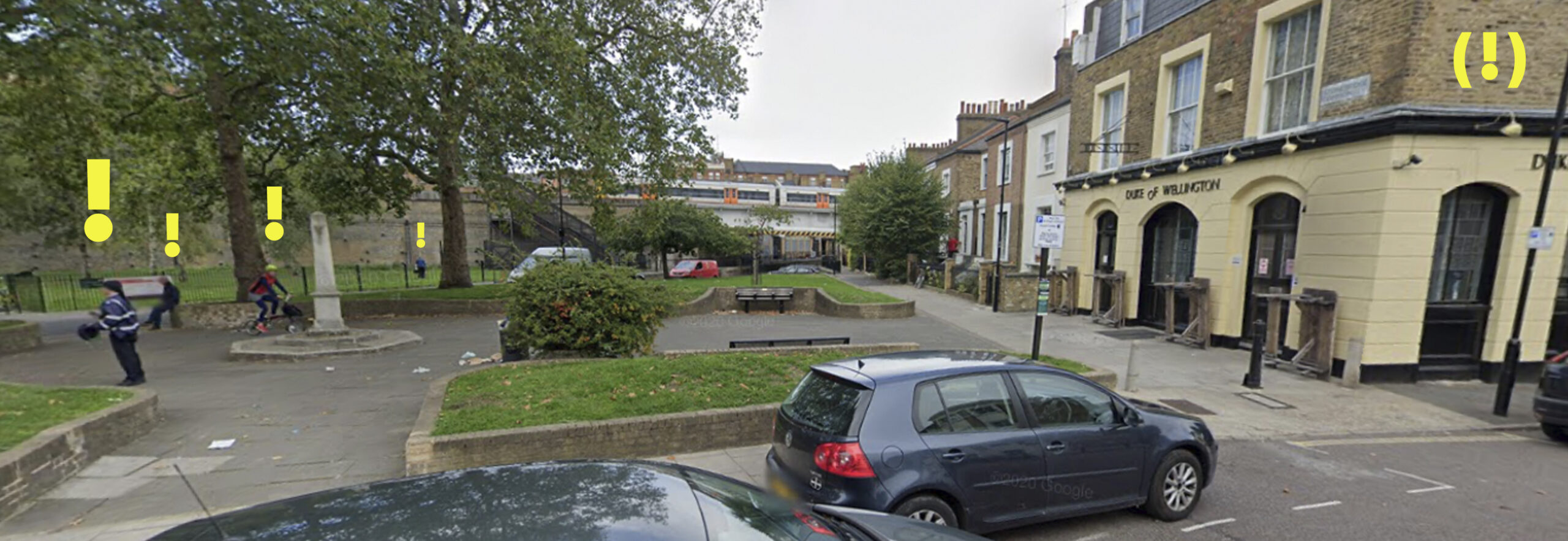
Recent Comments